While there is growing awareness in both doctor’s offices and health IT markets of the critical...
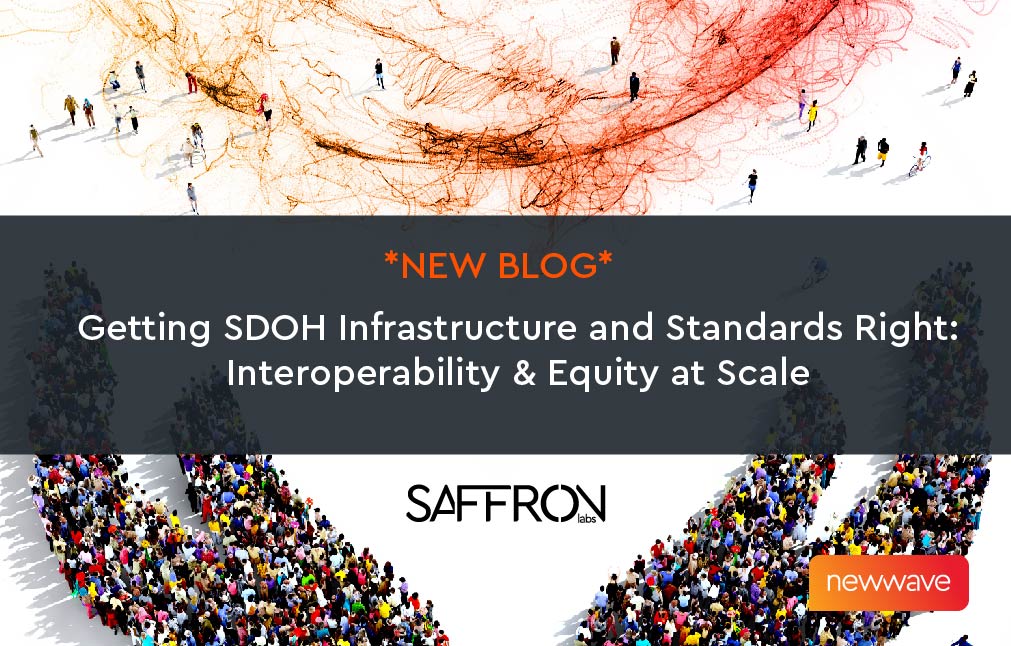
Back in March of this year, when we were still wrapping our minds around the severity and implications of the pandemic, a group of Senators and House members wrote to the leadership of the Department of Health and Human Services that the agency had a data problem. Among the failings of the federal response to COVID-19 was the inability of the government to account for the significant racial disparity in the impact of the virus in the collection and reporting of its data. Black and brown American’s are being devastated by the pandemic and that reality was not being made visible in the federal government’s data. The absence of that lived experience in the data also meant there was no policy response for those lives and that experience. The legislators observed in their letter:
It is critical that the federal government make a concerted effort to account for existing racial disparities in health care access and how persistent inequities may exacerbate these disparities in the weeks and months to come as our nation responds to this global health pandemic. We urge HHS to work with states, localities, and private labs to better collect data on health disparities as we continue to respond to this pandemic. To start, the CDC is currently failing to collect and publicly report on the racial and ethnic demographic information of patients tested for and affected by COVID-19.
And while the pandemic has made the federal government’s data “whiteness” problem visible, that problem is by no means limited to the new reality of the pandemic. A just published study by Olga Jarrín Montaner, assistant professor at Rutgers School of Nursing and Institute for Health, Health Care Policy, and Aging Research and Irina Grafova, assistant professor at Rutgers School of Public Health uncovers how whiteness is encoded in one of the largest health databases in the federal government: The Centers for Medicare and Medicaid Services Chonic Conditions Warehouse (CCW), a database NewWave has become deeply familiar with in our work with CMS.
The [CMS] administrative data contains two variables that are used for research and evaluation of health disparities: the enrollment database (EDB) beneficiary race code and the Research Triangle Institute (RTI) race code. … We found substantial variation between states in Medicare administrative data misclassification of self-identified Hispanic, Asian American/Pacific Islander, and American Indian/Alaska Native beneficiaries. Caution should be used when interpreting state-level health care disparities and minority health outcomes based on existing race variables contained in Medicare data sets. Self-reported race/ethnicity data collected during routine care of Medicare beneficiaries may be used to improve the accuracy of minority health and health disparities reporting and research.
The authors in their study “Beyond Black and White: Mapping Misclassification of Medicare Beneficiaries Race and Ethnicity”observe “[t]he inaccuracy of state-level data on Medicare beneficiaries’ race and ethnicity is staggering.”
Coded Bias
Yet, as much of the response to the present moment of increased awareness of structural racism shows, we live in a moment where change seems possible. When it comes to the erasure of race in federal data, the federal government and contractors like NewWave, who often execute the work of government, can do something. An anti-racist data science is possible. Writing in Medium, Emily Hadley, data scientist at RTI International offers such a path forward with her “5 Steps to Take as an Antiracist Data Scientist”
Data scientists are data stewards. We collect data, store data, transform data, visualize data, and ultimately impact how data are used. In our data-driven world, we have found ourselves with the responsibility to use data to tell stories and effect change.
But with this responsibility, it is not enough for us non-Black data scientists to simply not be racist. It is not enough for us to sit behind our computer screens to write code and feel angry but not take action after the deaths of George Floyd, Breonna Taylor, Ahmaud Arbery, and too many other Black individuals. It is not enough for us to acknowledge the racist systems that continue to exist in the United States but not actively do anything about them.
As Angela Davis said, “In a racist society, it is not enough to be non-racist. We must be antiracist.” Both non-racists and antiracists recognize that racism and white supremacy are wrong. Antiracists are those who take action to do something about it.
As non-Black data scientists, we must be antiracist data scientists. We must take responsibility for our power and privilege. We must confront the ways in which data and algorithms have been used to perpetuate racism, and eliminate racist decisions and algorithms in our own work. We must recognize that our field is lacking diversity (only ~3% of data scientists identify as Black) and contribute to pathways that change this. Being antiracist data scientists isn’t a one-time decision or something we will ever fully achieve, but instead a commitment we make each day, now and in the future, towards building a more equitable and just world.
In addition to the data scientists, engineers, developers, and technologists who often see themselves outside the mechanisms of racism, we must all assume responsibility of becoming part of the solution to achieve a more equitable and just world.